Objektklassifizierung mit APOC und SimpleITK-basierten Merkmalen#
Die Klassifizierung von Objekten kann die Extraktion benutzerdefinierter Merkmale beinhalten. Wir simulieren dieses Szenario, indem wir SimpleITK-basierte Merkmale verwenden, die in napari-simpleitk-image-processing verfügbar sind, und trainieren einen APOC table-row-classifier.
Siehe auch
from skimage.io import imread
from pyclesperanto_prototype import imshow, replace_intensities
from skimage.filters import threshold_otsu
from skimage.measure import label, regionprops
import numpy as np
import pandas as pd
import matplotlib.pyplot as plt
from napari_simpleitk_image_processing import label_statistics
import apoc
Unser Ausgangspunkt sind ein Bild, ein Labelbild und einige Ground-Truth-Annotationen. Die Annotation ist ebenfalls ein Labelbild, bei dem der Benutzer einfach Linien mit unterschiedlicher Intensität (Klasse) durch kleine Objekte, große Objekte und längliche Objekte gezeichnet hat.
# load and label data
image = imread('../../data/blobs.tif')
labels = label(image > threshold_otsu(image))
annotation = imread('../../data/label_annotation.tif')
# visualize
fig, ax = plt.subplots(1,3, figsize=(15,15))
imshow(image, plot=ax[0])
imshow(labels, plot=ax[1], labels=True)
imshow(image, plot=ax[2], continue_drawing=True)
imshow(annotation, plot=ax[2], alpha=0.7, labels=True)
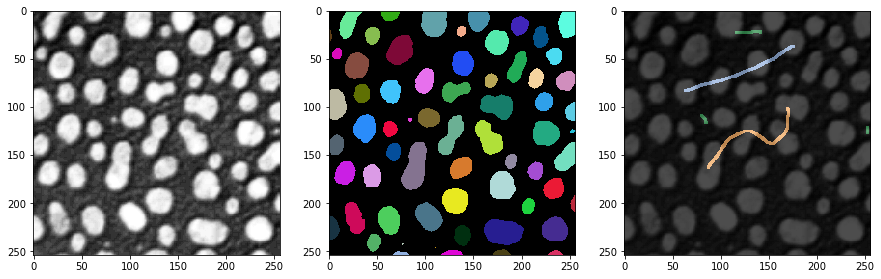
Merkmalsextraktion#
Der erste Schritt zur Klassifizierung von Objekten nach ihren Eigenschaften ist die Merkmalsextraktion. Wir werden dafür das skriptfähige napari-Plugin napari-simpleitk-image-processing verwenden.
statistics = label_statistics(image, labels, None, True, True, True, True, True, True)
statistics_table = pd.DataFrame(statistics)
statistics_table
label | maximum | mean | median | minimum | sigma | sum | variance | bbox_0 | bbox_1 | ... | number_of_pixels_on_border | perimeter | perimeter_on_border | perimeter_on_border_ratio | principal_axes0 | principal_axes1 | principal_axes2 | principal_axes3 | principal_moments0 | principal_moments1 | |
---|---|---|---|---|---|---|---|---|---|---|---|---|---|---|---|---|---|---|---|---|---|
0 | 1 | 232.0 | 190.854503 | 200.0 | 128.0 | 30.304925 | 82640.0 | 918.388504 | 10 | 0 | ... | 17 | 89.196525 | 17.0 | 0.190590 | 0.902586 | 0.430509 | -0.430509 | 0.902586 | 17.680049 | 76.376232 |
1 | 2 | 224.0 | 179.286486 | 184.0 | 128.0 | 21.883314 | 33168.0 | 478.879436 | 53 | 0 | ... | 21 | 53.456120 | 21.0 | 0.392846 | -0.051890 | -0.998653 | 0.998653 | -0.051890 | 8.708186 | 27.723954 |
2 | 3 | 248.0 | 205.617021 | 208.0 | 128.0 | 29.380812 | 135296.0 | 863.232099 | 95 | 0 | ... | 23 | 93.409370 | 23.0 | 0.246228 | 0.988608 | 0.150515 | -0.150515 | 0.988608 | 49.978765 | 57.049896 |
3 | 4 | 248.0 | 217.327189 | 232.0 | 128.0 | 36.061134 | 94320.0 | 1300.405402 | 144 | 0 | ... | 19 | 75.558902 | 19.0 | 0.251459 | 0.870813 | 0.491615 | -0.491615 | 0.870813 | 33.246984 | 37.624111 |
4 | 5 | 248.0 | 212.142558 | 224.0 | 128.0 | 29.904270 | 101192.0 | 894.265349 | 237 | 0 | ... | 39 | 82.127941 | 40.0 | 0.487045 | 0.998987 | 0.045005 | -0.045005 | 0.998987 | 24.584386 | 60.694273 |
... | ... | ... | ... | ... | ... | ... | ... | ... | ... | ... | ... | ... | ... | ... | ... | ... | ... | ... | ... | ... | ... |
59 | 60 | 128.0 | 128.000000 | 128.0 | 128.0 | 0.000000 | 128.0 | 0.000000 | 110 | 246 | ... | 0 | 2.681517 | 0.0 | 0.000000 | 1.000000 | 0.000000 | 0.000000 | 1.000000 | 0.000000 | 0.000000 |
60 | 61 | 248.0 | 183.407407 | 176.0 | 128.0 | 34.682048 | 14856.0 | 1202.844444 | 170 | 248 | ... | 19 | 41.294008 | 19.0 | 0.460115 | -0.005203 | -0.999986 | 0.999986 | -0.005203 | 2.190911 | 21.525901 |
61 | 62 | 216.0 | 181.511111 | 184.0 | 128.0 | 25.599001 | 16336.0 | 655.308864 | 116 | 249 | ... | 23 | 48.093086 | 23.0 | 0.478239 | -0.023708 | -0.999719 | 0.999719 | -0.023708 | 1.801689 | 31.523372 |
62 | 63 | 248.0 | 188.377358 | 184.0 | 128.0 | 38.799398 | 9984.0 | 1505.393324 | 227 | 249 | ... | 16 | 34.264893 | 16.0 | 0.466950 | 0.004852 | -0.999988 | 0.999988 | 0.004852 | 1.603845 | 13.711214 |
63 | 64 | 224.0 | 172.897959 | 176.0 | 128.0 | 28.743293 | 8472.0 | 826.176871 | 66 | 250 | ... | 17 | 35.375614 | 17.0 | 0.480557 | 0.022491 | -0.999747 | 0.999747 | 0.022491 | 0.923304 | 18.334505 |
64 rows × 33 columns
statistics_table.columns
Index(['label', 'maximum', 'mean', 'median', 'minimum', 'sigma', 'sum',
'variance', 'bbox_0', 'bbox_1', 'bbox_2', 'bbox_3', 'centroid_0',
'centroid_1', 'elongation', 'feret_diameter', 'flatness', 'roundness',
'equivalent_ellipsoid_diameter_0', 'equivalent_ellipsoid_diameter_1',
'equivalent_spherical_perimeter', 'equivalent_spherical_radius',
'number_of_pixels', 'number_of_pixels_on_border', 'perimeter',
'perimeter_on_border', 'perimeter_on_border_ratio', 'principal_axes0',
'principal_axes1', 'principal_axes2', 'principal_axes3',
'principal_moments0', 'principal_moments1'],
dtype='object')
table = statistics_table[['number_of_pixels','elongation']]
table
number_of_pixels | elongation | |
---|---|---|
0 | 433 | 2.078439 |
1 | 185 | 1.784283 |
2 | 658 | 1.068402 |
3 | 434 | 1.063793 |
4 | 477 | 1.571246 |
... | ... | ... |
59 | 1 | 0.000000 |
60 | 81 | 3.134500 |
61 | 90 | 4.182889 |
62 | 53 | 2.923862 |
63 | 49 | 4.456175 |
64 rows × 2 columns
Wir lesen auch die maximale Intensität jedes gelabelten Objekts aus der Ground-Truth-Annotation aus. Diese Werte dienen zum Training des Klassifikators. Einträge mit 0
entsprechen Objekten, die nicht annotiert wurden.
annotation_stats = regionprops(labels, intensity_image=annotation)
annotated_classes = np.asarray([s.max_intensity for s in annotation_stats])
print(annotated_classes)
[0. 0. 2. 0. 0. 0. 2. 0. 0. 0. 3. 0. 0. 0. 3. 0. 0. 3. 0. 0. 0. 3. 0. 0.
0. 0. 1. 0. 0. 0. 1. 2. 1. 0. 0. 2. 0. 1. 0. 0. 0. 0. 0. 0. 0. 0. 0. 0.
0. 0. 0. 0. 0. 0. 0. 0. 0. 0. 0. 0. 0. 0. 0. 0.]
Klassifikator-Training#
Als nächstes können wir den Random Forest Klassifikator trainieren. Er benötigt Tabellendaten zum Training und einen Ground-Truth-Vektor.
classifier_filename = 'table_row_classifier.cl'
classifier = apoc.TableRowClassifier(opencl_filename=classifier_filename, max_depth=2, num_ensembles=10)
classifier.train(table, annotated_classes)
Vorhersage#
Um einen Klassifikator auf den gesamten Datensatz oder einen anderen Datensatz anzuwenden, müssen wir sicherstellen, dass die Daten im gleichen Format vorliegen. Dies ist trivial, wenn wir den gleichen Datensatz analysieren, auf dem wir trainiert haben.
predicted_classes = classifier.predict(table)
predicted_classes
array([1, 1, 2, 3, 3, 3, 2, 3, 2, 1, 3, 3, 3, 2, 3, 1, 3, 3, 3, 3, 3, 3,
1, 3, 3, 3, 3, 1, 3, 3, 1, 2, 1, 2, 2, 3, 3, 1, 1, 3, 3, 3, 3, 2,
3, 2, 3, 2, 1, 3, 1, 3, 3, 1, 3, 3, 3, 3, 1, 2, 1, 1, 1, 1],
dtype=uint32)
Zu Dokumentationszwecken können wir die annotierte Klasse und die vorhergesagte Klasse in unsere Tabelle speichern. Hinweis: Wir machen dies nach dem Training, da sonst z.B. die Spalte
table
number_of_pixels | elongation | |
---|---|---|
0 | 433 | 2.078439 |
1 | 185 | 1.784283 |
2 | 658 | 1.068402 |
3 | 434 | 1.063793 |
4 | 477 | 1.571246 |
... | ... | ... |
59 | 1 | 0.000000 |
60 | 81 | 3.134500 |
61 | 90 | 4.182889 |
62 | 53 | 2.923862 |
63 | 49 | 4.456175 |
64 rows × 2 columns
table['annotated_class'] = annotated_classes
table['predicted_class'] = predicted_classes
table
/var/folders/p1/6svzckgd1y5906pfgm71fvmr0000gn/T/ipykernel_4463/2818530951.py:1: SettingWithCopyWarning:
A value is trying to be set on a copy of a slice from a DataFrame.
Try using .loc[row_indexer,col_indexer] = value instead
See the caveats in the documentation: https://pandas.pydata.org/pandas-docs/stable/user_guide/indexing.html#returning-a-view-versus-a-copy
table['annotated_class'] = annotated_classes
/var/folders/p1/6svzckgd1y5906pfgm71fvmr0000gn/T/ipykernel_4463/2818530951.py:2: SettingWithCopyWarning:
A value is trying to be set on a copy of a slice from a DataFrame.
Try using .loc[row_indexer,col_indexer] = value instead
See the caveats in the documentation: https://pandas.pydata.org/pandas-docs/stable/user_guide/indexing.html#returning-a-view-versus-a-copy
table['predicted_class'] = predicted_classes
number_of_pixels | elongation | annotated_class | predicted_class | |
---|---|---|---|---|
0 | 433 | 2.078439 | 0.0 | 1 |
1 | 185 | 1.784283 | 0.0 | 1 |
2 | 658 | 1.068402 | 2.0 | 2 |
3 | 434 | 1.063793 | 0.0 | 3 |
4 | 477 | 1.571246 | 0.0 | 3 |
... | ... | ... | ... | ... |
59 | 1 | 0.000000 | 0.0 | 2 |
60 | 81 | 3.134500 | 0.0 | 1 |
61 | 90 | 4.182889 | 0.0 | 1 |
62 | 53 | 2.923862 | 0.0 | 1 |
63 | 49 | 4.456175 | 0.0 | 1 |
64 rows × 4 columns
Darüber hinaus können wir denselben Vektor verwenden, um mit replace_intensities
ein class_image
zu erzeugen. Der Hintergrund und Objekte mit NaNs in Messungen werden in diesem Bild den Wert 0 haben.
# we add a 0 for the class of background at the beginning
predicted_classes_with_background = [0] + predicted_classes.tolist()
print(predicted_classes_with_background)
[0, 1, 1, 2, 3, 3, 3, 2, 3, 2, 1, 3, 3, 3, 2, 3, 1, 3, 3, 3, 3, 3, 3, 1, 3, 3, 3, 3, 1, 3, 3, 1, 2, 1, 2, 2, 3, 3, 1, 1, 3, 3, 3, 3, 2, 3, 2, 3, 2, 1, 3, 1, 3, 3, 1, 3, 3, 3, 3, 1, 2, 1, 1, 1, 1]
class_image = replace_intensities(labels, predicted_classes_with_background)
imshow(class_image, colorbar=True, colormap='jet', min_display_intensity=0)
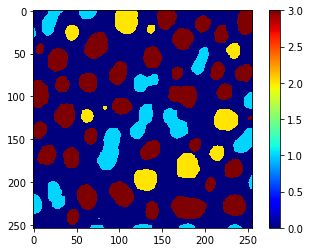