Pixelklassifizierung in Mehrkanal-Bildern#
APOC akzeptiert auch Listen von Bildern als Eingabe für Training und Vorhersage. Dies kann beispielsweise für die semantische Segmentierung in Mehrkanal-Bildern verwendet werden, die Zellkerne, Membranen und das dazwischenliegende Zytoplasma zeigen.
from skimage.data import cells3d
from skimage.io import imsave, imread
import napari
import numpy as np
import matplotlib.pyplot as plt
from pyclesperanto_prototype import imshow
image = cells3d()
image_ch1 = image[30, 0]
imshow(image_ch1)
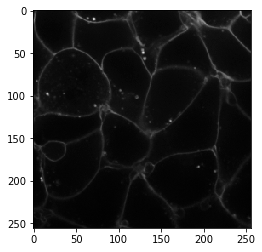
image_ch2 = image[30, 1]
imshow(image_ch2)
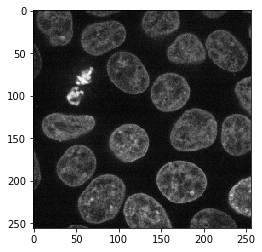
filename = '../../data/cells_annotation.tif'
annotation = imread(filename)
fix, axs = plt.subplots(2,2, figsize=(10,10))
imshow(image_ch1, plot=axs[0,0], colormap="Greens_r")
imshow(image_ch2, plot=axs[0,1], colormap="Purples_r")
imshow(annotation, labels=True, plot=axs[1,0])
imshow(image_ch1, continue_drawing=True, plot=axs[1,1], colormap="Greens_r", alpha=0.5)
imshow(image_ch2, continue_drawing=True, plot=axs[1,1], colormap="Purples_r", alpha=0.5)
imshow(annotation, labels=True, plot=axs[1,1], alpha=0.5)
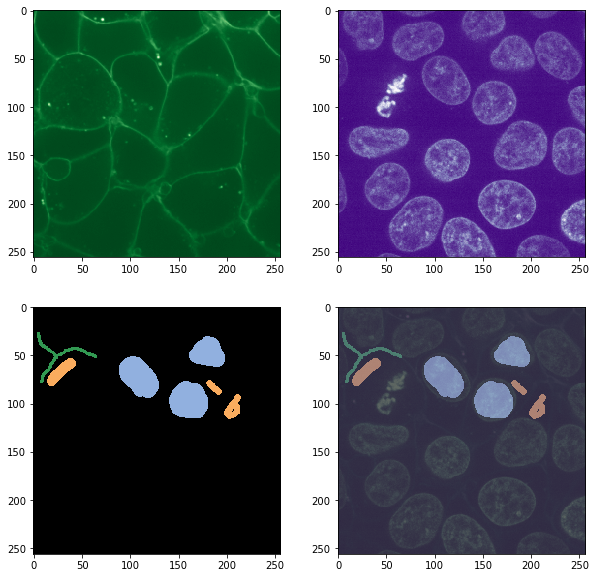
Training#
from apoc import PixelClassifier
# define features
features = "sobel_of_gaussian_blur=2 laplace_box_of_gaussian_blur=2 gaussian_blur=2 sobel_of_gaussian_blur=4 laplace_box_of_gaussian_blur=4 gaussian_blur=4"
# this is where the model will be saved
cl_filename = 'test.cl'
clf = PixelClassifier(opencl_filename=cl_filename)
clf.train(features=features, ground_truth=annotation, image=[image_ch1, image_ch2])
Vorhersage#
result = clf.predict(image=[image_ch1, image_ch2])
imshow(result, labels=True)
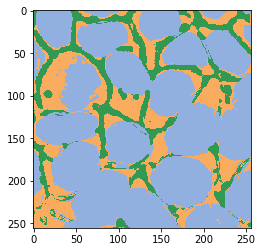